Blackboxing Law by Algorithm
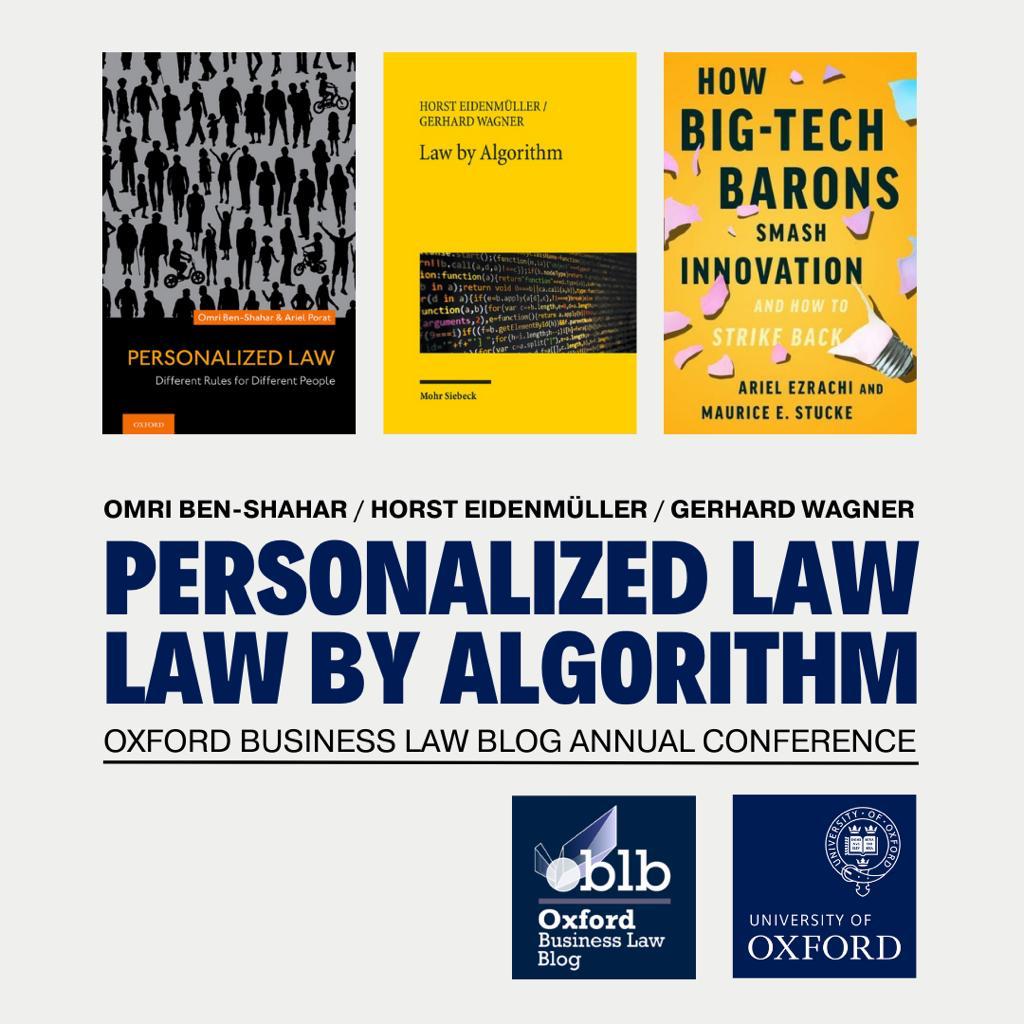
This post is part of a special series including contributions to the OBLB Annual Conference 2022 on ‘Personalized Law—Law by Algorithm’, held in Oxford on 16 June 2022. This post comes from Hans Christoph Grigoleit, who participated on the panel on ‘Law by Algorithm’.
Adapting a line by the ingenious pop-lyricist Paul Simon, there are probably 50 ways to leave the traditional paths of legal problem solving by making use of algorithms. However, it seems that the law lags behind other fields of society in realizing synergies resulting from the use of algorithms. In their book ‘Law by Algorithm’, Horst Eidenmüller and Gerhard Wagner accentuate this hesitance in a paradigmatic way: while the chapter on ‘Arbitration’ is optimistic regarding the use of algorithms in law (‘… nothing that fundamentally requires human control …’), the authors’ view turns much more pessimistic when trying to specify the perspective of the ‘digital judge’. Following up on this ambivalence, I would like to share some observations on where and why it is not so simple to bring together algorithms and legal problem solving.
1. (The problem is not on the empirical side—‘auxiliary service’) My first point is: we should clearly distinguish between the use of algorithms to solve empirical problems on the one side and the use of algorithms to apply the law and resolve normative issues on the other. By employing algorithms for empirical purposes, we do not apply ‘law by algorithm’. Rather, we make use of algorithms as an auxiliary service.
Many of the topics discussed in the discourse on legal algorithmization—and in the books that are the subject matter of this conference—refer to the empirical side of legal problem solving. In contrast to genuine normative problem solving, the use of algorithms as an empirical tool does not include drawing normative and final conclusions. It is auxiliary in that it resolves preliminary (empirical) questions which are ubiquitous in a normative setting.
Examples for such auxiliary services are algorithmic sentencing guidelines (referring to the probability of relapse), gathering of evidence (analysis of documents, etc), exploring damages claims of air passengers by reference to air traffic data (‘flight right’), adapting speed limits to traffic conditions, employing elements of ‘smart contracts’ (automatically adapting contract performance to digital input), etc.
Of course, there are overlaps and demarcation issues. However, generally speaking, the empirical use of algorithms is comparatively simple, as opposed to normative conclusions: where algorithmic tools are used for empirical purposes, the procedure does not categorically differ from making use of any other expert knowledge in a legal problem-solving-exercise. It is telling—and not surprising—that the auxiliary services are already a huge market, while ‘normative algorithms’ are not. Therefore, I will leave the field of empiricism and direct the focus of the following observations on using algorithms to resolve normative issues.
2. (The problem is inside the algorithms) It is common practice for legal scholars to discuss the effects of using algorithms without specifying how algorithms actually work. My second point is: we should learn more about algorithms and be more specific about their features when discussing their use for legal problem solving.
Admittedly, we know that algorithms operate in a certain order, formalized and restricted by the rules of logic. They reproduce the conditionalities that their designers have set up. They can be taught to learn, yet such a learning process is pre-set by conditionalities. Furthermore, we know their potential, that they can process data, we know that by processing data they can read minds and predict behavior and events—and all this in the most surprising ways. More specifically, we know that algorithms can—in the form of ontologies or knowledge graphs—organize complex subjects by making use of certain terms, which by their systematic order and their correlations allow drawing conclusions on the subject.
All this may sound a bit like the structure of legal reasoning. However, the sound may well be misleading. We do not yet have a good understanding of how exactly algorithms work when used to carry out specifically a normative exercise. This is because there are still very few individuals who are experts in the theoretical structure both of algorithms and legal reasoning. Consequently, algorithms used in a legal context can basically be qualified as a blackbox—and in the legal discourse they are routinely referred to as a blackbox.
While we see their potential outside the normative world, we must note two elementary limits in the context of normative decisions: (1) algorithms are designed by pre-set conditionalities; (2) the formal structure of algorithms is not expressed in natural language, and in this sense non-verbal. One may translate the findings of an algorithm in a verbal explanation. However, the more complex the algorithm, the more difficult it will be to verbalize its operation. And the easier it is to verbalize, the more dispensable it might be to use the algorithm.
3. (The problem is inside the legal reasoning) There is another—maybe even more important—reason for the knowledge gap concerning the delegation of normative exercises to algorithms: we may also be a bit inattentive about the structure of legal reasoning. Therefore, my third point is: we should become more accurate about legal reasoning, too.
a) (Purpose-evaluation) What we do know is that legal reasoning is not so much about terminology, about conditionalizing or about formal logic, but rather about the purposes that lie behind the terms. The relevant purposes are not explicitly and not exhaustively specified in legal sources. They must be established, evaluated and balanced with potential counter-purposes. This evaluation is partly normative—as it requires assumptions about the relative weight of purposes (in their context). But evaluating purposes is also partly empirical—as purposes relate to the real world, require assumptions about their intended (or: to be avoided) effects, to what extent they are accomplished, and what probabilities have to be taken into account.
b) (Distinguishing the legislation from the application level) This exercise of purpose-evaluation is an element of any legislative act. On the legislative level (parliament et al), purpose-evaluation is carried out in an abstract form. However, the purpose-evaluation cannot be comprehensively and exhaustively anticipated for all possible cases. Rather, it is the purpose-evaluation on the application level (the courts et al) that can and must take account of the infinite circumstances of the individual case and potential developments of the social and normative environment.
The context of each individual case may deviate significantly from the purpose-evaluation which was anticipated on the legislative level. Consequently, any application of the law must entail a purpose-evaluation which is supposed to be complementary to the evaluation that has already been carried out on the legislative level. In this sense, the purpose-evaluation on the application level is critical and innovative.
The theoretically infinite need for adjustment of the purpose-evaluation is equivalent to the assumption that no legal rule (or: sub-rule) is absolute. And no judge can apply the law in a purely conceptualized manner or as a law application machine.
c) (The uncertainty issue) With regard to most practical findings of applying the law, the process of purpose-evaluation is in fact quite simple and intersubjectively stable. He who concludes otherwise may be misled by the complexity which dominates the academic discourse, the causes célèbres and the public discussion.
This is to say that—within the scope of well-designed and established legal rules—the abstract purpose-evaluation supplied on the legislative level fits to most of the cases and allows drawing intersubjectively reliable conclusions. If this is ‘the case’, the need for specific purpose-evaluation may not even be conspicuous. This does not mean that in such a case evaluating the purpose of a statutory provision is dispensable. But, in simple cases, the implicit purpose-evaluation on the judicial level clearly does not go beyond that carried out on the legislative level.
However, there are obviously hard cases in which the purpose-evaluation does not warrant a clear result. With regard to the hard cases, we have to admit that we are confronted with uncertainties and ambiguities, and experience shows that legal reasoning does not always provide results which are intersubjectively reliable. The process of purpose-evaluation is still not yet understood in a fully rational or objective—or more modestly: in an intersubjectively reliable—way, even though it has been carried out for many decades. Therefore, in this specific sense, traditional legal reasoning may be qualified as a blackbox, too.
d) (Algorithms as a solution to the uncertainty issue) It is not likely that algorithms will solve the uncertainty issues of purpose-evaluation. In particular, it is not likely that algorithms will be successful in carrying out the critical or innovative function of purpose-evaluation. For this reason, it is also insufficient to make use of algorithms for predicting normative outcomes in the judicial system (as opposed to commercial uses, eg when insurance companies evaluate claims)—even though the results may be statistically promising. And, if one blackbox is supposed to be compared to another: The algorithmic one cannot (easily) be verbalized, whereas the input of a traditional purpose-evaluation can at least be verbalized (while the output cannot be intersubjectively proven).
4. (The problem is all inside your head) The uncertainty issue brings the factor of human intuition into play—and us back to the wisdom of Paul Simon. My fourth—and last—point is: The problem is all inside your head, and thus: we should also focus more on and explore the relevance of human intuition for legal problem solving.
Traditional law deals with the intuition issue in many ways: we expect normative decision-makers to feed their intuition only by the assumptions that are normatively relevant. Furthermore, we demand that the decision-maker substantiates his decision by providing his reasons. And we tend to control these reasons and the intuition risk by (what one may call) consensus tools: for instance, we refer normative decisions to panels (which may even be controlled by other panels), or we discuss normative issues in an open discourse, and we justify the products of our intuition by reference to ‘majority opinions’.
It is not inconceivable that at some point we will be able to replace human intuition by algorithmic intuition. The key issues will be whether (1) the process of purpose-evaluation can be carried out by algorithms and (2) we can provide similar safeguards for the uncertainties of intuition, in particular transparency of the relevant reasons.
However, at this juncture, it is hard to imagine that there will be acceptance for a comprehensive replacement of human intuition by algorithmic intuition. Rather, what we will see—and what we already see—is that human decision-makers use algorithmic tools to establish certain empirical assumptions, which are relevant in the normative context and for preparing the ground of applying human (and professional) intuition. This is why we are probably right to be reluctant to replace the human mind with the digital judge outright. We may rather stay with the former and continue to turn to the latter for some (and more) auxiliary services.
Hans Christoph Grigoleit holds the chair for civil law, commercial law, company law and private law theory at the University of Munich.
Share
YOU MAY ALSO BE INTERESTED IN
With the support of
