Global AI Governance – Part 3: A Fragmented Future and a Trump Twist
In two previous blog posts (here and here) we explained how foundation models are developed and adapted, how their characteristics lead to a difference between developer and deployer countries, and which strategies countries might adopt to have a say in the global, multidimensional AI governance chess game. This final post aims at describing the current status of global AI governance and sketch out possible ways forward. We also discuss the possible impact of the second Trump administration, succinctly explain which policies are currently discussed at the different levels of the value chain, and which challenges regulators will face in the global governance framework.
The Role of Pre-Deployment Guidance
To ensure compliance in practice, developers will want to have proper procedures in place to ensure the legality and safety of their products. At the moment several states try to provide guidance to developers to facilitate this task. The NIST AI Risk Management Framework, for instance, provides foundational guidance for assessing and mitigating risks throughout the lifecycle of AI systems. Additionally, large firms and other government agencies are creating responsible AI road maps (see eg here), associated tool kits and red teaming guidelines.
The NIST AI Risk Management Framework outlines several use case profiles that facilitate compliance with regulatory requirements. By adhering to these profiles, developers can significantly reduce the likelihood of legal and ethical issues arising after deployment. This, in turn, fosters trust and reliability in AI technologies. As further AI laws get enacted, these AI implementation frameworks will play an increasingly crucial role in ensuring compliance in the at times complex regulatory landscape created by global AI governance.
Significantly, pre-deployment testing routines not only play a significant role in the AI Act (Articles 9, 15 and 55), but would also have become mandatory for particularly powerful, future AI models under the Californian SB 1047. While that bill, passed by the California legislature, was eventually vetoed by the governor, it shows that the trajectory of global AI regulation clearly leads towards more pre-deployment testing and interventions, particularly for the most powerful models.
The Open-Source Conundrum
While the art of pre-deployment testing has not yet developed into mature science, the AI governance landscape is rendered even more complex by one of the thorniest problems in AI governance: open-source AI models. A spectrum of approaches are available to access foundation models ranging from completely open-source to entirely closed models, encompassing hosted access, API access, and the open sourcing of parameters, information on architecture, training data, and learning mechanisms. These models present a regulatory puzzle that policymakers will have to find a workable solution for.
The term ‘open-source’ in commercial AI circles might not align with its definition in AI governance frameworks. Take the EU AI Act, for instance. To qualify as ‘open-source’ and enjoy certain exemptions, a model needs to bare it all:
- Publicly available parameters (including weights)
- Information on architecture and model usage
- Free access, usage, modification, and distribution
Some models we casually label as ‘open-source,’ might not make the cut under these stringent criteria. The OLMo 7B model from the Allen Institute is an example of a model that meets all the requirements. Even though the AI Act does not compel the disclosure of all training data (Rec. 102), the extensive requirements (parameters, architecture, usage) still mean that a number of models colloquially called ‘open source,’ such as the ones by Mistral and Meta, may not qualify for the Act’s open-source exemption if only weights and the model are published for free.
Under the AI Act, open-source models benefit from some limited exemptions: They are exempted from transparency obligations for foundation models (Art 53(2) AI Act) and from specific documentation duties in the AI value chain (Art 25(4) AI Act). However, these exemptions do not apply to general-purpose models with systemic risk, ie, particularly powerful foundation models (Art 51 AI Act). The idea behind this legal architecture is: if information on the model is divulged as part of the open sourcing process, legal obligations in this direction are obsolete. However, for the most capable models, the AI Act still intends to ensure that all of the critical information mandated in its Annex XI (eg, on training data; biases; and energy consumption) is indeed made available to the general public. However, if open-source models are integrated into high-risk AI risk systems, the rules governing those systems do apply (Art 9 et seqq. AI Act); similarly, if open-source models do interact with human subjects, they have to abide by the transparency provisions of Art 50 AI Act (eg, notice about AI use and deep fakes). In the US, to the best of our knowledge, there have been findings and recommendations from advisory committees such as the National AI Advisory Committee and the NTIA (an agency of the Department of commerce) (see here) but no specific laws to address the benefits and risks of ‘open source’ models. In the US the recommendation has been to monitor these dual use, open weight foundation models to determine if changes in policy are required.
Regulating for Frontier Open-Source Models
More generally, policymakers are performing a delicate balancing act between fostering innovation and mitigating risks. Open-source models can drive competition, research, and AI adoption. But these models share many risks with closed models and can be harder to control.
As we venture into the realm of frontier and future AI models—think GPT-6 and beyond—the stakes get even higher. Hence, the debate in technical and policy circles is heating up, as these models can be easily stripped of safeguards and misused by malicious actors for nefarious purposes. A specific example is the question of watermarks and data provenance. The content provenance alliance (c2Pa.org) whose membership includes all the major model developers in the US of closed and open source models as well as other important players such as Adobe, Microsoft and others have developed data provenance standards and watermarking technology (e.g, google SynthID) to track how data has been transformed and modified and produced by AI. However, there are a number of models (small and large) created in the US and elsewhere in the world that are not part of the C2PA. Malicious users can create content without adherence to C2PA data provenance and watermarking and it is possible that some of these labels can be stripped using open source technology. So while private sector led solutions have significant value, in the absence of a legal framework, governance will fall short.
Recent studies suggest that the marginal risk of open-source models compared to just using internet access and Google search isn't very significant... yet. But as models become more powerful, agentic, and capable of executing complex reasoning tasks, the game changes.
The lesson for international AI governance is clear: we need adaptive, capability-based regulations. As models surpass certain capability thresholds—determined through rigorous pre-deployment safety testing—they may need to shift further towards the closed end of the spectrum.
The future might look something like this: privileged access for researchers to study these powerful models, but not fully deploying them as open-source to prevent easy circumvention of safety features.
The open-source conundrum adds yet another layer to our developer-deployer chess game. It's not just about who creates or uses the AI anymore; it's about how accessible and modifiable these powerful tools should be.
Managing Risks after Deployment
After deploying a model, new challenges emerge. The focus shifts to the continuous monitoring of the model and reporting adverse events. One way to address this challenge, which is also proposed by the US National AI Advisory Committee, is the use of AI Computer Emergency Response Teams (AI CERTs). The CERT concept stems from traditional software development where CERTs are groups of experts that provide immediate assistance in the case of cyber security incidents. Similarly, AI CERTs could mitigate the effects of a post-deployment security risk caused by a foundation model (there already is an AI incident monitor hosted by the OECD). Fixing vulnerabilities in AI models causes, however, its own set of challenges. When issues can only be fixed by retraining a model, this can lead to extensive costs. To commit stakeholders to transparency and accountability, as well as to get them to collaborate on these issues, the law needs to provide for an effective enforcement mechanism.
Evaluating risks stemming from AI is still a work in progress. At the moment some innovative approaches for AI evaluation are being developed (see here and here). How exactly they will be identified and mitigated in practice can therefore not be estimated with certainty. To ensure that the mitigation strategies can move from theory to practice, it is therefore important that this research receives continuous support.
Developing Global AI Standards
We have already stressed that standardization can play a crucial role in AI governance and lead to a certain degree of globalization at the deployer level of regulation. The United States High Level Advisory Body on AI has recently published its final Report on 'Governing AI for Humanity' calling for a 'coherent effort' in global AI governance. The EU Code of Practice for general-purpose AI is underway. Standardization can be one way to achieve additional coherence in this regard. Consensus among states often converges on softer and more general principles of AI regulation. This consensus has led to the adoption of some abstract commitments towards ethical, secure, inclusive and globally beneficial AI in the Global Digital Compact. Stakeholders, and states, will now have to operationalize these abstract commitments into concrete policies.
Technical standards can be a focal point for policymakers when acting on their abstract commitment. They are expert advice on how to mitigate risks posed by AI and legislators will know that orienting regulations towards these standards will decrease trade barriers. In case states reach a consensus on the importance of standards they might even decide on a rule akin to that of Art 40 of the AI Act: Compliance with the NIST framework, ISO and CEN/CENELEC standards could be the benchmark for minimum regulation, and crosswalks should be created between these standards, to harmonize global efforts to manage AI risks.
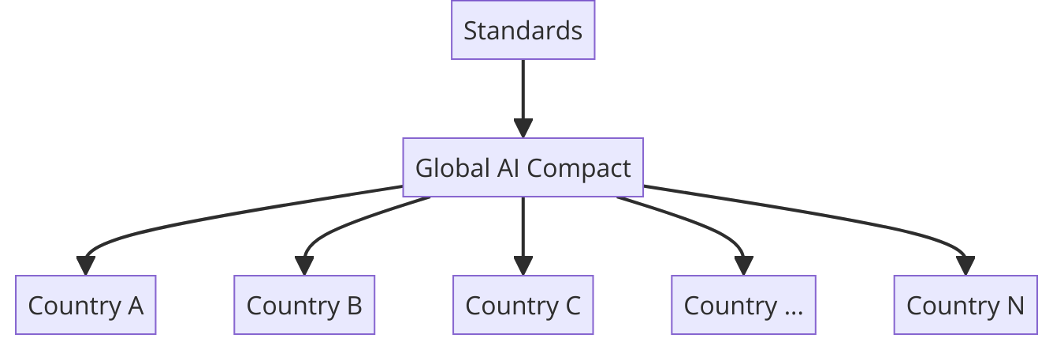
AI Governance under Trump
The 2024 election of Donald Trump harbingers a significant shift in US AI policy, with the incoming administration poised to reshape the regulatory landscape both at home and potentially even globally. Trump has pledged to repeal President Biden's AI Executive Order, which he characterized as ‘dangerous’ and hindering AI innovation. While the campaign promises suggest a wholesale rollback, the reality is likely to be more nuanced. A review of the 2016 Trump administration AI executive orders and rulemaking highlight areas of shared emphasis with the Biden Administration executive order pertaining both to innovation as well as to elements deemed crucial for the US geopolitical stance, for example related to national security and cybersecurity.
The new administration is expected, however, to otherwise pursue a markedly different approach to AI governance from its predecessor. Most likely, it will prioritize innovation with an eye towards national competitiveness with a commitment to protecting civil liberties vs. an overt focus on civil rights protections best articulated in the Blueprint for an AI Bill of Rights. Unlike Biden's emphasis on algorithmic fairness, AI safety and potential AI harms, Trump's policy is likely to focus on accelerating AI development, particularly in national security and defense contexts. This may prompt states, particularly those led by Democrats, to redouble their own efforts at AI regulation, adding to the patchwork of state-level rules already puzzling AI developers. His heterodox defense approach could potentially lower barriers for smaller, more innovative technology firms to enter the defense and cybersecurity markets. This approach will likely also include protectionist measures aimed at preventing China from benefiting from US AI advancements, with a strong emphasis on maintaining technological superiority.
As a consequence, internationally, the shift in US AI policy could have significant implications for global AI governance. While the Biden administration had been actively working to establish international frameworks and voluntary commitments for AI development, the Trump administration may take a more unilateral approach. The focus is expected to be on promoting American AI leadership, potentially through increased investment in R&D and a more aggressive stance on technology competition with China, despite cautionary voices about its effectiveness. This approach would indeed contrast with, and pose a significant challenge to, the sketched efforts to build multilateral consensus for global AI coordination and standards.
Conclusion
The journey from policy to practice in international AI governance is long and winding. We have shown that the AI value chain can lead to a complex chain of regulations. This complexity can cause challenges in existing AI legislation. The characteristic of foundation models to be adapted for a specific use-case after development blurs the line between developers and deployers in the AI Act that will – given the current state of AI governance – need to be resolved by the courts. Based on this characteristic of foundation model development one can apply a theoretical distinction between entire countries: developer countries and deployer countries.
The possibility to effectively regulate the big developers of AI models will likely remain the privilege of few powerful countries. Regulating the deployment of these models in the national market, by contrast, remains possible for many non-developer countries – while a hierarchy in regulatory power persists among the deployer countries. To maintain their regulatory power, states should aim at avoiding the accumulation of market power in the deployer markets for individual actors. Deployer states can furthermore try to exert additional influence over developers by trying to establish international standards for AI.
When implementing their local policies states should focus on several key aspects. They should review their ‘vertical’ regulations and make sure that they do not run counter to their efforts of regulating AI. In addition, their regulatory success will depend on their enforcement capacities. This requires proper funding and the accumulation of technical knowledge in the country.
In our view it is crucial to establish international standards and cooperation among global stakeholders to foster a safe, secure, and equitable AI ecosystem. Ultimately, we should not forget that the pursuit of effective AI governance is not only about mitigating risks. It is also about harnessing the transformative potential of AI for some of the most urgent challenges in our societies.
The 2024 Trump administration may, however, represent a significant inflection point in US AI policy. The administration's approach is likely to prioritize innovation over civil rights protections, to which US states may respond with further AI rules of their own. The incoming administration is also poised to emphasize technological competitiveness and national security. This geopolitical strategy adopts a more aggressive stance towards technological competition, particularly with China, potentially reshaping global AI governance dynamics.
Philipp Hacker is Professor for Law and Ethics of the Digital Society, European New School of Digital Studies.
Ramayya Krishnan is Dean, Heinz College of Information Systems and Public Policy and Ruth F. Cooper Professor Of Management Science And Information Systems, Carnegie Mellon University.
Marco Mauer is Researcher, European University Institute.
Share
YOU MAY ALSO BE INTERESTED IN
With the support of
