Law and Autonomous Systems Series: Algorithmic Credit Scoring and the Regulation of Consumer Credit Markets
Posted
Time to read
Recent advances in machine learning (ML) and Big Data techniques have enabled the development of more sophisticated, automated consumer credit scoring models—a trend referred to as ‘algorithmic credit scoring’ in recognition of the increasing reliance on computer (particularly ML) algorithms. This post examines the rise of algorithmic credit scoring, and considers its implications for the regulation of consumer creditworthiness assessment and consumer credit markets more broadly. Although it focuses on the UK, the technological developments and regulatory implications discussed are of wider relevance to other jurisdictions.
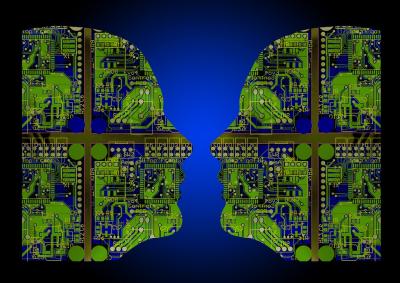
Consumer Credit Regulation and the Objectives of Creditworthiness Assessment
Pursuant to UK consumer credit regulation, credit providers are required to assess the ‘creditworthiness’ of borrowers. This entails an assessment of both the credit risk to the lender (ie the probability of default by the borrower and loss given default), as well as the affordability of the credit for the borrower. From a regulatory perspective, the credit risk requirement seeks to ensure that capital is allocated efficiently—to the most valuable projects in the least-cost manner—and to minimize adverse spillover effects (financial and real) due to systemic non-performing loans. Of course, lenders also have a commercial incentive to manage credit risk in order to mitigate their own losses due to non-performing loans.
The requirement to assess affordability seeks to mitigate possible financial difficulties to the borrower due to over-indebtedness. It also limits the ability of unscrupulous lenders to intentionally exploit the behavioural and cognitive weaknesses of less financially literate borrowers by selling them unaffordable credit products. Such rent-seeking behaviour by lenders is inefficient, as effort is expended simply to bring about a transfer of wealth from borrowers to lenders, without increasing economic output. Moreover, it can lead to undesirable distributional outcomes, as lower-income and lesser educated borrowers are typically more susceptible to such forms of exploitation.
The Rise of Algorithmic Credit Scoring
Credit scoring entails the use of statistical techniques to model and predict a borrower’s creditworthiness. Traditional credit scoring models draw conclusions primarily from patterns in past credit performance and financial account transaction data, gleaned from multiple borrowers. This approach reflects both demonstrated statistical correlation between credit history and the likely credit risk of a borrower, as well as traditional limits on lenders’ access to non-financial, and/or non-credit data about borrowers. However, it often results in borrowers with sparse or non-existent credit histories (so called ‘thin-file’ or ‘no-file’ borrowers) scoring poorly, or being deemed un-scorable. These borrowers are frequently denied credit by mainstream lenders, and are forced to rely on ‘payday lenders’, or informal, unregulated sources of credit—often at punitive interest rates and exposing them to abusive lending practices.
The growth of algorithmic credit scoring could address some of these limitations. There are two partially overlapping dimensions of change represented by algorithmic credit scoring: the first relates to the use of a larger volume and variety of data (‘alternative’ data); the second relates to the use of more sophisticated techniques to analyse the data. Regarding the first dimension, alternative data includes both non-credit, financial data (for example, direct data on rental and mobile phone bill payments), as well as non-credit, non-financial data—for example, ‘social’ data captured from consumers’ social media networks, and ‘behavioural’ data about consumers’ habits and preferences.
The second and more recent development embodied by algorithmic credit scoring is the use of ML techniques to analyse the data. This in turn impacts the first dimension: the types of data that can be used. Significantly, ML algorithms can parse very large volumes of data—especially, raw, unstructured, high-dimensional, and/or anonymized data—to find correlations that could be (more) relevant to predicting a borrower’s creditworthiness. Notably, ML algorithms can more accurately capture non-linear relationships in the data, as well as reflect changes in the population and environment by ‘learning’ from new training data. A form of ML called ‘deep learning’, using multi-layer neural networks, has shown particular promise in analysing unstructured and high-dimensional data.
Impact of Algorithmic Credit Scoring on Consumer Credit Markets
Expanding the number and types of measured variables, and employing ML techniques, thus allows for a more detailed, multi-dimensional observation of a borrower’s characteristics that can be used to estimate their creditworthiness. This is particularly important for thin file and no-file borrowers, who may present an acceptable credit risk despite not having any conventional, financial credit data to support this assessment. As such, by enabling more accurate creditworthiness assessment, algorithmic credit scoring stands to enhance the efficiency of consumer credit markets.
Furthermore, by widening access to credit for thin-file and no-file borrowers, algorithmic credit scoring can help to redress extant distributional and fairness concerns in consumer credit markets, given that these borrowers are more likely to be from low-income, less educated and ethnic minority backgrounds. Algorithmic credit scoring could also reduce the scope for unfairness due to ‘statistical discrimination’. By increasing the observability of non-protected characteristics relevant to a borrower’s creditworthiness, the incentive for lenders to rely on conventionally more observable yet protected characteristics, such as sex or race, as statistical proxies for creditworthiness, should reduce.
Conversely, however, there is a risk that the opacity and complexity of certain ML approaches could make it more difficult to pre-empt or verify ex post whether the system has (inadvertently) facilitated unlawful discrimination, by relying on protected characteristics, or proxies for them, in reaching a credit decision. Relatedly, biases in the training, validation and/or test data used to build ML scoring models could perpetuate past discrimination in lending. For example, an ML model trained on data from a predominantly white population could result in bias against lending to non-white populations. Likewise, spurious correlations in these datasets can lead to inaccurate (and potentially unfair) predictions when the model is applied to new, ‘out of sample’ data.
Algorithmic credit scoring could furthermore become a source of inefficiency and unfairness in consumer credit markets if it is used by lenders to more effectively exploit the cognitive and behavioural limitations of borrowers. Inter alia, a lender could use behavioural insights derived from algorithmic credit scoring to more precisely target a borrower, or profiled groups of borrowers, with unfavourable credit offers at moments of extreme vulnerability. This could increase the chance that a borrower reflexively agrees to an unfavourable contract, without carefully reviewing its terms or shopping around for a better offer.
The question arises whether these same technologies deployed in the hands of borrowers could attenuate such risks. For example, ML and Big Data are already being used to build highly personalised web plugins and mobile apps that seek to counteract behavioural biases, and ‘nudge’ consumers into making better financial decisions. However, whether these applications can be effective in this way depends on the extent to which they are adopted by consumers. Less financially literate consumers are less likely to understand the value of these solutions, in order to avail of them in the first instance. Moreover, to the extent that these solutions do not fully replace financial decision-making by consumers, the latter, compromised by behavioural and cognitive weaknesses, could simply ignore the advice offered by the relevant app.
More importantly, it is questionable whether these applications will be able to fully overcome the informational and behavioural advantage that lenders have over borrowers, and which they use to exploit borrowers. In particular, lenders enjoy privileged access to aggregate financial transaction data (conventional ‘credit data’) and product use patterns gleaned from multiple transactions with borrowers over time, that could be difficult for third party consumer-helping platforms to substitute.
Regulatory Challenges and Opportunities of Algorithmic Credit Scoring
Algorithmic credit scoring thus presents itself as a double-edged sword. On the one hand, it stands to benefit consumer credit markets, inter alia, by improving the accuracy of creditworthiness assessment and thereby widening access to credit from mainstream lenders. On the other hand, it could generate new sources of inefficiency and unfairness through the exploitation of consumers’ cognitive and behavioural weaknesses, and unlawful discrimination. To the extent that the market, in the form of consumer-helping applications, is unable to offer a complete solution to these risks, consideration must be given to whether and if so how government-backed regulation should be strengthened.
As a general matter, the principles and conduct-based approach of the UK consumer credit regulatory regime gives regulators flexibility to respond dynamically to the use of new and fast evolving technologies, such as algorithmic credit scoring, by market participants. In particular, the principles that firms must ‘treat customers fairly’, act with ‘due care, skill and diligence’, and ensure that product marketing is ‘clear, fair and not misleading’, provide a broad legal basis for regulators to respond to potential exploitation and discrimination against consumers through the use of algorithmic credit scoring, as well as for firms to design appropriate systems and controls in order to achieve the outcomes enshrined in these principles.
To complement their dialogue with firms under the principles-based approach, regulators could themselves make greater use of ML and Big Data techniques to more directly detect, understand and remedy undesirable behaviour by market participants. This includes, for example, empirically assessing how consumers respond to particular forms of product marketing, in order to ascertain whether it is ‘clear, fair and not misleading’. These findings can be used to inform regulatory changes, for example, mandating greater personalisation of information disclosure by firms, or requiring firms to adjust the consumer choice architecture in a more targeted way (for example, changing the default settings on their website or app to mitigate common consumer mistakes).
Likewise, firms could be required to put in place more robust governance and oversight arrangements specifically relating to their ML systems and processes, including algorithmic credit scoring systems. Inter alia, this could encompass procedures for data quality verification, as well as continuous model feedback testing, cross-validation and auditing to mitigate data overfitting and algorithmic bias risks. These procedures should build on the data protection auditing, certification, impact assessment and data protection ‘by design and default’ provisions under the EU General Data Protection Regulation (GDPR), the new data protection regime in the EU.
Indeed, cross-sectoral data protection regulation provides an important additional mechanism for mitigating potential discriminatory and unfair treatment of credit consumers due to the processing of their personal data through algorithmic credit scoring. Inter alia, the overarching principles that guide the GDPR could, if interpreted strictly, significantly restrict the potential for firms to abuse consumers’ personal data. These include, in particular, the principles of ‘purpose limitation’—requiring personal data to be collected only for ‘specified, explicit, and legitimate purposes and not further processed in a manner that is incompatible with those purposes’—and ‘data minimisation’, requiring personal data to be ‘adequate, relevant and limited to what is necessary in relation to the purpose for which they are processed’.
The GDPR furthermore expands the rights of data subjects to control the use of their data, including a potentially broader right to receive ‘meaningful information about the logic involved’ in automated decision-making (the so-called ‘right to explanation’). An expansive interpretation of this right (and corresponding duty) by credit providers and/or regulators—for example, requiring credit providers to provide an ex post explanation to individual borrowers of the specific reasons underlying each credit decision—could better support borrowers in challenging discriminatory and unfair credit decisions.
On the other hand, an expansive interpretation of data protection principles, rights and duties risks undermining the potential efficiency and fairness gains from algorithmic credit scoring. With respect to the principle of purpose limitation, as this post has highlighted, algorithmic credit scoring largely relies on repurposing data to uncover hidden insights about a borrower’s creditworthiness. Likewise, a more onerous ‘right to explanation’ could be undesirable if it restricts firms to using statistical techniques that are simpler and more ‘explainable’, yet less effective in assessing creditworthiness.
Conclusion
Algorithmic credit scoring, and the Big Data and ML technologies underlying it, present both benefits and risks for consumer credit markets. This post has argued that the broadly principles and conduct-based approach of UK consumer credit regulation provides the flexibility necessary for regulators and market participants to respond dynamically to these new technological risks. This approach could be enhanced through the introduction of more robust product oversight and governance requirements for firms in relation to their use of ML systems and processes. Supervisory authorities could also themselves make greater use of ML and Big Data techniques in order to strengthen their supervision of consumer credit firms. Finally, cross-sectoral data protection regulation, recently updated in the EU under the GDPR, offers an important avenue to mitigate risks to consumers arising from the use of their personal data. However, the interpretation of this regime in the consumer finance context needs to be carefully calibrated, so as not to also inhibit the potential benefits of new technological applications such as algorithmic credit scoring, and Big Data and ML more generally.
Nikita Aggarwal reads for a DPhil in Law at Brasenose College, University of Oxford.
Share
YOU MAY ALSO BE INTERESTED IN
With the support of
