All Souls Blog: “Predictive Algorithms in the Justice System: The implications of neutrality logics”
Posted
Time to read
Dr Pamela Ugwudike is an Associate Professor of Criminology and the Director of Research at the Department of Sociology, Social Policy and Criminology, University of Southampton. She completed her master’s degree in Criminology and her PhD at Swansea University. Her research is mainly focused on the interplay of digital technology and criminal justice. Currently, she takes part in several research-related projects that investigate digital predictive technologies and outlets of algorithmic bias in policing and penal services, as well as digital governance and regulatory solutions for law enforcement agencies. In general, she is interested in the experiences of AI technologies and how they transform crucial elements of the social sphere in particular politics, equality, and justice.
Her All Souls Seminar focused on the intersection of data bias, knowledge production, and criminal justice. During her presentation, she draws on insights from the sociology of race in order to demonstrate the implications of race neutrality logics in predictive algorithm models. Prof Ugwudike, particularly problematises the conceptualisation of data-driven algorithms as neutral. In law enforcement, algorithms are supposedly race-neutral with no overt references to race. Yet, according to Prof Ugwudike, structural conditions such as racial bias can sustain regardless of apparent race neutrality. She makes two distinct arguments. The first draws on the underlying structural condition of power discretion that is available to the creators of the algorithm. The second demonstrates the implications of the assumption that algorithms are risk-neutral. Prof Ugwudike illuminates that data-driven algorithms can be understood as an element that is producing and perpetuating racially biased predictions. Therefore, it is crucial to think about how data-driven criminal justice maintains and simultaneously transforms the structural power inequalities.
Algorithms and the neutrality logic in the criminal justice system
According to Prof Ugwudike, the term algorithm can be best described as a set of code rules or instructions that perform certain functions or tasks which are used in the justice system to predict crime risk. Predictive algorithms are applied in key areas of the criminal justice decision-making for instance in policing, sentencing, probation and in prison. The underlying premise for this sort of risk-focused approach to making criminal justice decisions is the so-called risk neutrality. Yet, there is growing evidence that they can produce racially biased predictions. The presumption of risk neutrality is what some scholars within the sociology of race – according to Prof Ugwudike – conceptualise as a liberal ideology, which is based on what she describes as a set of risk neutrality logics. Prof Ugwudike demonstrates that implications of this liberal race neutrality ideology can mask the structural conditions that sustain bias and prejudice in the justice system.
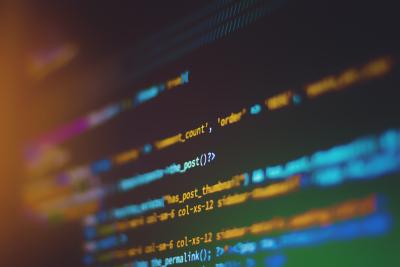
Masking discretionary decision-making
These logics are reproduced through various structural power discretions that are available to those who create or design these algorithms. While the use of discretionary decision-making can sometimes be useful in specific institutional contexts, a problem with the usage of discretion is that it is able to introduce bias into decision-making and infuse the design of predictive algorithms with racial bias, even when the algorithms appear to be ‘risk-neutral’. Thus, the made predictions mirror the data that was used to produce them. If Black suspects are arrested at a higher rate than white suspects in the real world, they will have a higher rate of predicted arrest. This means they will also have higher risk scores on average, and a larger percentage of them will be labelled as ‘high-risk’. Thus, the predictive model can ultimately produce certain variables that can be considered a proxy for race. For Prof Ugwudike, this highlights the potential danger for apparently ‘risk-neutral’ algorithms to reproduce the biases that are embedded in the data on which they rely for predictions.
The digital divide and the implications of neutrality logics
According to Prof Ugwudike, three sociological concepts are useful for understanding the structural problem of an uneven distribution of algorithmic biases: digital capital, digital exclusion and the digital divide. From a sociological perspective, digital capital or information capital can be defined as the ability to acquire the resources that are required for gaining the full benefits of technology such as predictive algorithms. This includes the knowledge, skills or capital to obtain the power and ability to create the technologies. This conceptualisation of digital capitalism draws our attention to the asymmetric power relationship that exists between the people whose lives are affected by the algorithms because they are labelled as ‘risky’. The concept of digital exclusion is also relevant here, as certain groups are assigned with little or no digital capital and are thus likely to be affected by digital exclusion. This is because they do not have the required digital capital for creating these technologies, so they have little to no influence over the processes of creating these tools of prediction. Thus, their values, preferences and circumstances on how the algorithm will impact their lives, is not considered when the tools are created. According to Prof Ugwudike, this highlights what some sociologists described as the so-called digital divide. On one side of the divide are those who are able to utilise their discretionary powers to produce digital technologies like predictive algorithms, while on the other side of the digital divide, are those whose lives are most likely to be affected by the outputs of the technologies.
Solving the issue of predictive algorithms in the criminal justice system
Having in mind the inherently negative characteristics of predictive algorithms in the criminal justice context, Prof Ugwudike developed several solutions for bettering the technology of crime prediction. One possible remedy is to democratise the processes of creating algorithms. This involves permitting the community, whose lives are often negatively affected by the algorithms to take part in the choices as well as decisions that go into the process of creating the algorithm. Moreover, Prof Ugwudike proposes obligatory internal and external audits of their tools to check for potential biases. In order to do so, legal frameworks ought to be introduced to govern key phases of creating or designing algorithms, including the process of selecting the data that the algorithms will use for prediction. This could potentially enhance transparency and accountability of law enforcement agencies. According to Prof Ugwudike, a special regulatory body could be established to audit algorithms and monitor how they are employed.
Predictive algorithms and their prescribed race neutrality logics certainly mask the structural conditions that make it possible to infiltrate criminal justice decision-making processes. In her presentation, Prof Ugwudike suggests that neutrality logics also encompass potential conditions of biases in the processes of selecting data predictors and recidivism variables, which ignore underlying digital inequalities. She proposes that there is a need to create different approaches to democratise and build a new legal framework to address the issue of injustice in predictive algorithms. Prof Ugwudike has spent several years studying the application of digital technology in the criminal justice realm. Her presentation provides an innovative and empirically sound contribution to the controversial debate surrounding predictive criminology and its relationship to race, discrimination and inequality.
Blog post by Anna Kahlisch
Current MSc Student in Criminology and Criminal Justice at the Centre for Criminology, The University of Oxford

Keywords:
Share
YOU MAY ALSO BE INTERESTED IN